Program at a Glance
Program
Business Analytics, MS
Format
Online, Hybrid, On Campus
Total Units
36
Requirements
No GMAT or
GRE Required
Program Overview
Big Data for Big Business
Data Analytics is a field of explosive growth and burgeoning opportunity. As organizations scramble to find qualified employees, savvy professionals are looking to cash in on the demand by establishing themselves in the field. Golden Gate University’s Master of Science in Business Analytics (MSBA) program opens the door to this lucrative world by providing courses that are designed for application of analytics to address real-world business challenges.
The Master of Science in Business Analytics presents students with an understanding of the many possibilities for applying data analytics to business problems. Data analytics, and the implications of this strategic discipline, give practitioners new opportunities for discovering insights that can support the strategic goals and decision making of the organization. The discipline has grown so fast that it is impossible to address all of its elements, so this degree should be viewed as a “toolkit” of statistical and analytic theory, processes, tools, and techniques, which can be integrated into the business depending on the discipline and needed outcomes.
The MSBA is relevant to multiple audiences, including: the business manager charged with using data analytics to derive value from data and/or leveraging analytics teams to get that value; the subject matter expert in a business discipline charged with using analytics on the job; the budding business analytics data scientist requiring understanding of a myriad of data analytics tools; and the IT professional responsible for supporting the analytics infrastructure and addressing issues of data security, privacy and ethics. Students completing the MSBA will have earned 39 units including three units of graduate statistics.
Admission Requirements
- Applicants should hold a bachelor’s degree from a regionally accredited US institution or the equivalent from a recognized foreign (outside the US) institution, and provide official transcripts.
- Applicants whose native language is not English must meet the English Language Proficiency Admission Requirements.
- Applicants are required to submit a statement of purpose and a resume.
- Applicants with a bachelor’s degree GPA below 3.0 may also submit master’s degree transcript for consideration.
Proficiency Requirements
Math Proficiency
Intermediate Algebra (Math 20); See Graduate Mathematics Proficiency Requirement for more information.
Writing Proficiency
Students are expected to possess proficiency in writing to ensure they can be successful in their course of study. Students may meet this requirement by satisfying one of the screening criteria listed under Graduate Writing Proficiency Requirement.
Curriculum
The Master of Science in Business Analytics requires completion of 36 units in the graduate program and a 3-unit graduate statistics course. Foundation program courses may be waived in accordance with university policy regarding course waivers. The foundation program must be met before starting any degree courses, except MSBA 300.
The foundation program provides the groundwork for the advanced program courses and concentration courses.
(Must earn a “B” or better before starting advanced program courses.)
Students may select any six of the following courses:
Learning Outcomes
Graduates of the Master of Science in Business Analytics degree program will have the knowledge and skills to:
- Explain the differences between structured and unstructured data, aligning each with appropriate business applications.
- Articulate and align with corporate performance, the complexities of data management, including organizational structures, data policy, data governance, data ownership, and data strategies.
- Explain and give examples of the three analytic disciplines of descriptive, predictive, and prescriptive (optimization).
- Identify the different kinds of tools used in optimization and simulation and explain their appropriate usage in the work environment.
- Identify and explain the steps of the CRISP-DM process model.
- Anticipate challenges to data security, privacy and ethics, recommending reasonable solutions to issues when they
occur. - Recognize the challenges of Big Data and describe the use of supporting technologies.
- Use visual outcomes of analytics to communicate effective messages to members of the business community.
- Describe the different approaches to machine learning, demonstrating application of the most common algorithms.
- Explain Natural Language Processing, identifying potential uses and challenges.
- Interpret and analyze individual business problems, selecting the best analytic approach and appropriate tools for extracting value from the data.
- Promote data quality by effectively acquiring, cleansing, and organizing data for analysis.
- Plan and implement the use of self-service analytics in the workplace, addressing the challenges of stand-alone implementations.
- Explain the influence that AI has on business processes and execution.
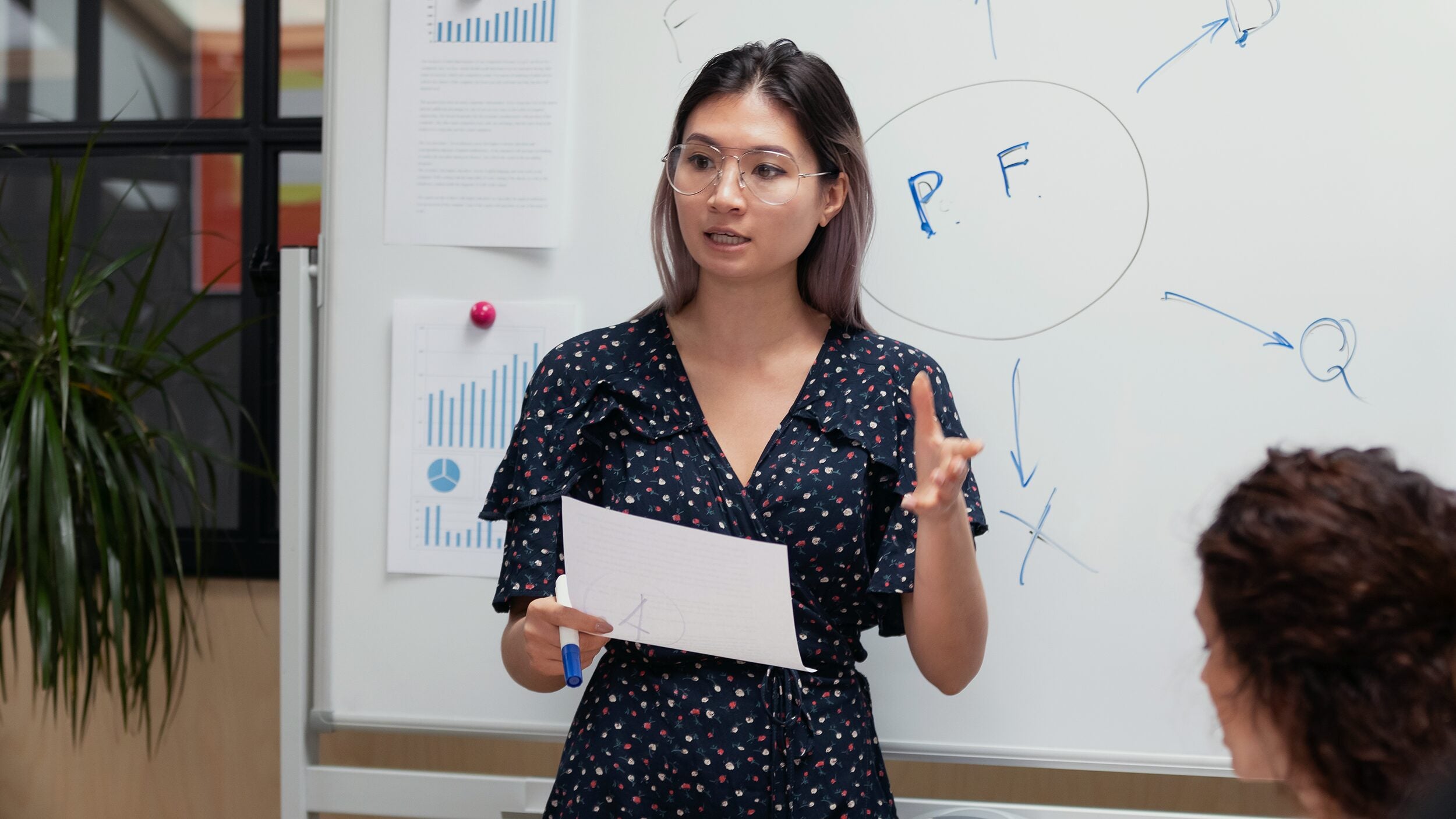